Introduction
What counts as viable knowledge and “good” scholarship varies considerably from discipline to discipline–and for every scholar working within the context of the digital humanities (or any other humanities field with interdisciplinary inclinations) this eventually means running up against the snarly question of qualitative vs. quantitative data. Despite our reliance on textual evidence, carefully constructed arguments, and a working knowledge of critical theory, as literary scholars most of the data we work with is highly qualitative in nature–indeed, the term “data” itself would be roundly objected to by many of our colleagues! For a sociologically-inflected project like What Every1 Says (WE1S), studying public discourse on the humanities at large data scales, this means tackling the challenge of providing our qualitative data with a transparent, rigorous, and reproducible methodology for interpreting vast amounts of subjective text, from which we then develop hypotheses, tools, and guidelines for a variety of audiences. For our multi-million article corpus, this methodology is topic modeling and the interpretation protocols our team developed to effectively read them. On scales too small to effectively topic model, however (in the tens or scores of articles) this methodology is hand-coding, based on a Constructivist Grounded Theory approach.
The purpose of this essay is to explain how our team used a modified Constructivist Grounded Theory approach to develop a hand-codebook for the WE1S project, meant to both compliment and provide a slightly different perspective on our larger corpus and central questions. However, before explaining in more detail what Constructivist Grounded Theory is, how we used it, and why, a few basic definitions are in order. To a digital humanist (and, indeed, to most people at all familiar with digital technology), “coding” is generally understood to mean “writing computer programs”. But this is not what hand-coding means. Rather, in this context, “coding” can be better understood as labeling; that is to say, as a way of “tagging” large amounts of written data using a stable set of codes, or signifiers, that can be referred to and refined later on. For example, given the sentence “The humanities teach a variety of “soft” skills that are hard to quantify, but are essential to the workplace”, I might use the label “Employability and Income”, as well as “Humanities and Liberal Arts Advocacy” (see our completed codebook below). Or, for example, if I am reading an article that says “Governor so-and-so has decided to cut the state college’s liberal arts budget by 20%, citing the need for a STEM-oriented future”, I might use the label “Policy and Politics” as well as “Funding”. While this approach makes little sense on a very small scale (say, with one article), its purpose quickly becomes clear when you are dealing with multiple articles and/or working in a team, from which you are attempting to extrapolate a stable and replicable set of relating factors across multiple documents and readings. In other words, hand-coding is about seeing beyond individual details and detecting larger patterns across many different texts and contexts. As you read more and more, your codes (or labels) become more stable, and you begin seeing what kinds of patterns come up again and again. Most importantly, hand-coding is an iterative process, and depends on multiple “passes” in order to generate any sort of reliable set of codes–first, you are working on a sentence-by-sentence basis, but then you continuously “zoom out” to larger scales, repeating the steps (see “Constructivist Grounded Theory” below) on larger chunks of text, and eventually coding your codes and looking for “organic” patterns in the data. In this way, hand-coding is a lot like topic modeling–just on a smaller and more human-intuitive scale.
The key to successful hand-coding is twofold–first, and most importantly, you must not begin with a predetermined set of codes with which you would like to label your data. Although you almost certainly have some kind of central question or interest in mind, it is important to proceed inductively rather than deductively–in other words, don’t impose your own expectations upon the data before giving it a chance to “speak for itself”. While the ultimate purpose of developing a codebook is to create a stable set of referents for your corpus, this can only occur after many iterations and reconsiderations of your initial codes–having a predetermined set of labels not only leads to sloppy scholarship and confirmation bias, but also can prevent you from seeing important and interesting patterns that you would not have guessed existed.
Second, in contrast to the commonly understood steps of the scientific method (or, for that matter, writing an essay within the humanities), Constructivist Grounded Theory works best when you do not begin with a hypothesis–rather, your analysis should be “grounded” fully in the data itself. Although it is difficult not to make guesses or jump to initial conclusions about meaning, it is important to concentrate on accurate labeling rather than evaluating. This is another way in which hand-coding turns out to be quite similar to many Digital Humanities projects working with large data scales. Just as your codebook gradually refines itself to best fit your data, any meaning you attach to your codes, any hypotheses you choose to test, and any conclusions you eventually reach must come later, after “living in” the data for some time.
Constructivist Grounded Theory
To carry out our exercises and develop our WE1S codebook, we used a modified version of Constructivist Grounded Theory framework, developed by sociologist Kathy Charmaz. Grounded theory methodologies were originally developed in the context of sociology by Barney G. Glaser (Charmaz’s teacher) and Anselm L. Strauss, as a response to an increasing turn toward positivist conceptions of the scientific method, and an insistence on empirical verifiability within the field. While acknowledging the problematics of the participant observer “lone researcher” model, which tends to over-focuses on the narrative case study, Glaser and Strauss nevertheless maintained that qualitative data is an essential part of studying human discourse–a fact which the social sciences must not lose sight of while trying to garner approval by modeling themselves after their “hard” counterparts. A human studying other humans cannot help but co-create the complex realities that they observe, either through direct participation or via an inevitably personal interpretive lens: “[P]ositivist methods [in the social sciences] assumed an unbiased and passive observer who collected facts but did not participate in creating them, the separation of facts from values, the existence of an external world separate from scientific observers and their methods…Glaser and Strauss essentially joined epistemological critique with practical guidelines for action” (6-7). Charmaz further refined Glaser and Strauss’s work through implementing the constructivist turn into the method, emphasizing the fact that qualitative data is always based on discourses that are necessarily partial, contingent, and situated (236). “Constructivist grounded theory [both] highlights the flexibility of the method and resists mechanical applications of it”, creating a methodology in which theory is arrived at inductively rather than deductively (13).
In other words, social context–which necessarily includes the sorts of questions that the researcher is interested in–cannot be abstracted away through false claims of neutrality, or glossed over via top-down, grand theories of human behavior. Rather, it is possible for qualitative data sets to be analyzed in a strategic and systematically robust way (“grounded” in the firm foundation of the data itself), while at the same time acknowledging that social realities are complex, multifaceted processes beyond any individual’s control, rather than a set of objective structures waiting to be “discovered” (11).
Charmaz’s methodology is best understood as a series of strategies, which diverse researchers can utilize–in part or in whole–in a variety of empirical situations and disciplines involving qualitative data. At its core, it depends on iterative processes of data collection and analysis, using comparative methods and drawing on data to develop new conceptual categories rather than reading it “through” a preexisting theoretical framework. “Grounded theory concepts can travel within and beyond their disciplinary origins…you can adopt and adapt them to solve varied problems and to conduct diverse studies” (16). Thus, it is uniquely suited to our purposes, and provides both a counterweight and a guide to interpreting the machine reading methods we are employing at large data scales for the WE1S project.
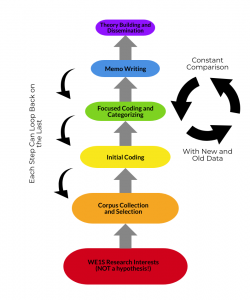
This chart has been adapted from Kathy Charmaz’s Constructing Grounded Theory, 2nd ed (2014).
Constructivist Grounded theory consists of a series of iterative steps, which are not linear but tend to loop back on themselves and one another as necessary:
1) Development/Operationalization of a Research Question
This often shifts and changes as more data comes to light, or new patterns emerge–much like how, in WE1S, we choose which models to scrutinize based on the question, just as we shuffle the way we ask the question so that the models can answer it meaningfully. As coding commences, the researcher’s incomplete understanding of the data continues to raise questions and refine the properties of the codes, which in turn leads to revision of the research question(s).
2) Data Collection
In Charmaz’s case, this consisted almost entirely of transcribed interviews with research subjects; in ours, the vast majority of our data comes from newspaper and journal articles that are published in the public sphere, with only a relatively tiny amount being tied directly to human subject survey results–nevertheless, the qualitative nature of both our and Charmaz’s respective data sets remains clear.
3) Initial Coding
Before any codes are established, and intial coding exercise entails through each document and annotating its margins–paraphrasing, condensing, and otherwise recording initial impressions. During this stage, no attempt is made to either refine or conglomerate codes–this is a “first pass”. Ideally, Charmaz would have us do this with each sentence, and then return to intial coding at different stages throughout the research process, as a way of seeing old data sets with “fresh eyes”. Due to limited time and humanpower, we restricted our initial coding to a single meeting, with no guidelines on how many codes we should produce per sentence or paragraph. However, in all subsequent coding sessions, researchers were encouraged not just to utilize/expand/redefine the existing codebook, but also think of redundancies, gaps, and other issues with the codebook, which often resulted in new codes. However, unlike Charmaz, we did NOT subject each piece of data to an initial coding procedure–this iterative step makes far more sense when working with transcribed individual interviews than it does when working with hundreds of newspaper articles, most of which share the same publisher (The New York Times), and are thus subject to the same general editing standards and guidelines. For our purposes, shifting more of our attention to the next step–focused coding–was preferable (see “Purpose of Hand-Coding”).
4) Focused Coding and Categorizing
This stage of constructivist grounded theory is perhaps best described as “coding the codes”; that is, looking for codes that tend to do the same thing, descriptive gaps, and other broad-ranging (but nonobvious) patterns in the intitial codes. It is an exercise in building a codebook that is both robust enough to describe and analyze much of the data, while at the same time being specific enough to that data set to remain meaningful and incisive (i.e., the aforementioned “Goldilocks Zone” between too narrow and too wide). We practiced this kind of focused coding during the remainder of our meetings as we continued to build the codebook, although we remained open to the induction of new codes and the deletion of old ones as we continued our theoretical sampling of the data (see “Hand Coding Methodology and Process”).
5) Memo Writing
This stage consists of writing short, “memo-length” observations, ideas, and musings to yourself as the grounded theory process continues to expand and grow. The idea behind memo-writing is threefold: first, so that the thoughts of a moment are not lost or buried in the larger slough of data analysis as you go; second, so that the memos themselves can be compared/constrasted in yet another, higher-level version of focused coding and pattern discovery; and finally, so that the particularly useful/insightful memos can then become part of the eventual report or essay that you publish on the research project itself. Ideally, Charmaz’s memo-writing happens continuously during data collection, initial coding, focused coding, and eventual theory-building. It also represents what is perhaps our greatest departure from Charmaz’s methods; we largely skipped this entire step, since (for our purposes) the robust codebook itself (as opposed to a published paper) was the goal for our hand-coding exercises. Still, it is arguable that our version of “memoing” consisted of the roundtable reports and subsequent discussions that the individuals on our research teams had with one another after each round of coding–these were the primary impetus for changing and revising the codebook itself, and resulted in emergent codes each time we came together.
6) Theory Building and Dissemination (Publication)
This stage involves putting memos together to evolve an overarching theory or framework analysis via which to understand your data. Recall that Charmaz’s method was developed by and for sociology, which largely adheres to the “lone researcher” model preferred by many of the social sciences (at least when it comes to fieldwork with human subjects). The greatest strength of including theory building in the process itself is that it discourages the researcher from forcing the data to fit the contours of a preexisting theory or framework on the one hand, or freewheeling speculation on the other–rather, it encourages a fresh analysis that is nevertheless solidly “grounded” (hence its name) in the data set that the researcher has been collecting and analyzing, generally for the space of years. While for Charmaz, including this particular module in the blueprints of her method makes perfect sense, for our project much of the “theory building”, and the publications/tools that will arise therin, come from the analysis of the models themselves, for which our team’s codebook can serve as a starting point and guiding framework.
It should be clear by this point that Charmaz’s method is both modular and adaptable to various situations, research questions, and disciplines that deal with qualitative data sets: be they classical literature, transcribed interviews, or hundreds of newspaper articles. In miniature, it does what WE1S is attempting to do at much larger scales through our engagement with topic models. Constructivist grounded theory, like topic modeling depends on constant and iterative comparison. The advantage of human reading and coding small parts of our data set is that this comparison can happen not just between different pieces of data, but also in between stages; as codes and categories form, the initial research question also morphs and changes as the researcher codes new data, recodes old data, codes the codes, writes down her observations as they occur, scrutinizes them alongside the codebook, identifies gaps, and engages in theoretical sampling of more data, all the while working toward theory building that goes beyond the initial question set. Constructivist Grounded Theory is particularly well suited to research within the humanities, insofar as it acknowledges both the positionality of the researcher and the sociocultural limitations of a given study. It does not attempt to create a grand theory for ALL situations, but rather a straightforward analysis of a particularly situated question, from which others may be extrapolated (19).
Getting Started
From February-May of 2019, during the second year of the WE1S project, the “Primary Corpus Collection and Analysis” Team spearheaded a series of “hand-coding” exercises on a number of small, human-readable corpora of news and social media articles. These were selected from our 1BC (“One Big Corpus”) of all collected work to date. Obviously, the scope and range of our selected articles represent only a fraction of a percentage of our main project’s scale; however, we did our best to select a range of article types and subjects, generally focusing on 15-20 articles per session. The codebook was developed iteratively over the course of five team discussions, including one coordinated hand-coding exercise across three collection teams. For the sake of simplicity, our team selected all of the articles for the first four sessions from the New York Times, which represents a signficant portion of our 1BC as well as a “gold standard” form major mainstream news sources.
After our initial crafting of the codebook, we expanded our exercises to include article sets containing keywords other than “humanities”, both in order to see how well our codebook was fitted to our particular corpus, as well as to determine whether or not it was viable to collect data on other keywords (see “Purpose of Hand-Coding”). Finally, we worked with the other collection and analysis teams to code sections of their subcorpora using our codebook, gathering practical suggestions on how we might modify and refine the final document to ensure both robustness and specificity. For the cross-team coding exercise, we branched out to include articles from a number of team-specific news sources other than the New York Times (see “Hand-Coding Methodology and Process”), in order to further expand and refine our codebook’s useability.
Although our codebook was initially envisioned as a simple way to tell whether or not it was viable to collect on keywords beyond “humanities” and “liberal arts” (for which the answer was mostly negative; see “Our Hand-Coding Methodology and Process”), we did produce a valuable deliverable for the project in the form of our codebook, specially suited to our 1BC (see “Outcomes”). It allowed us to articulate several “About” codes that continuously appear in discourse regarding the humanities, which has the potential to furnish both language and guidance when interpreting topic models and/or reporting results. Finally, I propose that the hand-coding process provides an easily graspable inroad, both for participants in the project and its eventual beneficiaries, to understanding what topic modeling does, how it works, and why it is uniquely suited to qualitative data sets such as public discourse. Although topic modelling obviously differs from hand-coding insofar as it is concerned with word collocation rather than subjects per se, as well as an exponentially massive difference in scale, the two processes nevertheless rely on similar strategies of iterative refinement, of finding non-obvious patterns and similarities, and working with a larger number of documents than a normal human, close-reading, would be able to utilize in a non-anecdotal or piecemeal manner.
Our Hand-Coding Methodology and Process
To develop our WE1S codebook, we utilized a modified version of Kathy Charmaz’s Grounded Theory methodology (Charmaz 2014 (2006)). For the initial coding exercise, our team (consisting at the time of 7 members) all read and annotated the same 15 articles, and then met to compare notes about our codes. A “code” refers to an abbreviation, word, or short phrase that serves as shorthand for a part of the article’s content. Our “codebook” refers to a collection of finished codes (those which proved both versatile and specific) suited to our corpus, along with their definitions (see “Outcomes”).
For the purposes of comparing grounded theory methodology to topic modelling, one might usefully (though the analogy is not perfect) think of a “code” as a topic that a given article falls into. Just as the same key, high-weight word can be part of a number of topics in a standard DFR browser, in hand-coding the same article can (and usually does) have several different descriptive codes attached to it. In topic-modelling, the machine is doing at the level of word collocation what, in grounded theory, the human is doing at the level of discourse patterns (see “Purpose of Hand-Coding” for a fuller discussion of this).
Ideally, when developing an initial set of codes, a reader will closely code the first set of articles they encounter (one code per paragraph, or even per sentence), and then “zoom out” to look for patterns and combinations from there. Because we were working with a fraction of a massive corpus, of which we could not possible hand-code the entirety, we focused on producing somewhat general codes (3-6 “about” codes per article) from the beginning, rather than spending significant time at the sentence or paragraph level. Additionally, since we were working with published articles rather than typed interview transcriptions (for which Charmaz originally developed her method; see “Constructivist Grounded Theory”), we quickly agreed that we needed at least three kinds of code to properly identify each article in context:
- Genre codes (signifying discourse form, such as feature article, advice/opinion, social media, etc)
- Rhetorical codes (reflecting the tone or agenda of an article)
- “About” codes (reflecting the content of the article itself)
An ideal coding exercise for a single article, then, will result in one genre identifer code, at least one (but not more than three) rhetorical code(s), and as many “about” codes as necessary–to accurately but not painstakingly describe its content. Because I had the most experience with utilizing Grounded Theory methodology, and had initially suggested it to my teammates, I was put in chart of maintaining and iteratively revising a single codebook document, which included writing detailed definitions for each code. Because we were working with a tiny sliver of a much larger corpus, we used a different set of articles for each iterative exercise, and eventually (for the third and fourth meetings), a different article set for each team member. Our fifth meeting involved cross-coding exercises with the two other collection/analysis teams, using our codebook and their subcorpora.
After our initial meeting to draft a rough but working version of our codebook, in subsequent meetings each team member looked at a different set of 15-20 articles, experimenting with and refining the codes we had developed to look for gaps, gratuitous repetitions, and overly general codes. A well-crafted codebook is necessarily tailored to the corpus it serves, and strives for the so-called “Goldilocks Zone” of being neither too general nor too specific. Codes that continuously occur together (for example “policy” and “politics”) can often be grouped together into a single entity. In contrast, a code that is being used continuously on a number of differing articles, “working overtime” as it were, probably needs to be split into smaller and more specific entities. When non-sequiturs and outliers occur, they should be noted, but may ultimately be dismissed if they prove to be unique. Grounded Theory hand-coding methodology is looking for rules and patterns, rather than exceptions.
After two iterations of working on a general codebook, our team split up; each member searched for 15-20 articles containing one of a number of different keywords (such as “history”, “philosophy”, and “science”) in addition to “humanities”, in order to test and iteratively refine the codebook for a wider range of articles within our 1BC. Our fourth meeting included attempting to use the refined codebook on articles containing these keywords, but that did NOT contain the word “humanities”. We determined that our codebook needed some (but not substantive) refinement, and that collecting on other keywords (with the possible exceptions of “literature” and “art history”) was not a good strategy given the larger goals of WE1S and the functionality of topic modeling. The fact that our codebook “broke” for most articles that didn’t contain the word “humanities” is a positive sign–it indicated that our codebook, which we had already proven to be robust by iteratively refining it over the course of 100+ articles, was also tailored specifically to the needs of the WE1S “humanities” corpus; that is to say, flexible enough to provide a descriptive baseline for our 1BC, but not so general as to fit other data sets.
For our fifth and final exercise, we engaged in cross-team coding, headed by myself and Leila Stegemoeller for the “Students and the Humanities” Team, and by Jamal Russell for the “Diversity and Inclusion” Team. In both of these instances, we selected a small corpus of articles (20-25) from those teams’ major collection sources, including The Chronicle of Higher Education, University Wire sources, and UCSB’s own Daily Nexus, for the “Students” Team, and an assortment of publications from ProQuest’s “GenderWatch” and “Ethnic Newswatch” Databases for the “Diversity and Inclusion” Team. This exercise resulted in a very fruitful discussion about generality vs. robustness, a number of revised definitions in the working codebook, and productively identified a few gaps (such as “K-12” and “Student Life”) which we had not noted during our initial exercises.
Purpose of Hand-Coding
As noted earlier, hand-coding can be thought of as a sort of “human topic-modeling”, in which the human researcher is looking for discourse patterns and tendencies that would not be apparent by simply summarizing a given text, or even by close-reading a small handful of texts. Just as the DFR browser “baggifies” the words from thousands or millions of articles and then iteratively sorts them into topics (or categories) based on collocation tendencies, the human researcher begins hand-coding by creating a shorthand code for every detail (ideally, for each sentence), and then returns to the beginning of the text to refine these codes at the two-sentence level (looking for similarities, repetitions, non sequiturs, etc), and so on and so forth, eventually resulting in a set of codes that are neither too specific nor too general, that can usefully describe and analyze the corpus at hand on small scales. Our human-developed codebook (see “Outcomes”) can also serve as a baseline or beginning reference point for using topic models to answer specific research questions, as we will begin to do in earnest in Summer 2019.This is why the iterative method of developing a codebook is essential–taking the first, rough-hewn attempts at a codebook and applying it over and over to different data sets from the same corpus, re(de)fining and revising along the way, the outcome is a series of robust codes or discourse patterns that is both empirically transparent (it leaves a processual “paper-trail”) and dependent on the qualitative interpretation and close-reading practices valued by the humanities.
This last observation emerged as something of a boon from our original goal with our hand-coding exercises, which was far more modest. Initially, we envisioned hand-coding as a way of determining whether or not it was viable to mass-collect articles with keywords other than “humanities” and “liberal arts” (and, for our projected UK corpus, “the arts”). With the exception of a few relatively uncommon and highly discipline-specific words such as “art history”, “English literature”, and (to a lesser extent) “literature”, the results of this experiment were negative. The fact that our humanities-specific codebook “broke” when confronted with keywords other than “humanities”, however, is actually a good sign–it indicates that there are public discourse patterns specific to mentions of the humanities that tend not to occur elsewhere. Were our codebook to work equally well with any handful of articles, it would indicate that either a) our codebook was so astoundingly general as to be, practically speaking, useless, or b) that there is no significant discursal difference between a corpus of articles that mentions “humanities” and one that does not–which would effectively and devastatingly founder the central goal of the WE1S project. Seeing as how neither of these things happened, we are left with a robust codebook that serves as a baseline “aboutness” indicator, as well as a reassurring “sanity check” that all our work to date has not been for naught.
Outcomes
There is no such thing as a perfect codebook, and both the range of our data and the number of (re)iterations that we practiced were far fewer than Charmaz would have recommended. However, since the codebook was envisioned as a guide to keyword collection and (later) as a general touchstone to provide vocabulary and guidance toward initial research questions (See “Purpose of Hand-Coding”), the level of granularity and refinement that we did manage to achieve–combined with the fact that analysis of the topic models themselves is the primary purpose of WE1S–was deemed sufficient to generally identify the major “aboutness” of the humanities as they appear in public discourse, as well as the broad patterns of overarching rhetoric that tends to accompany them.
Below is a reproduction of our finished codebook, as of June 2019:
Genre Codes: | |
Feature | “Regular” articles. This is the majority of our corpus |
Blog | Similar to feature; metadata will often determine this as a separate genre |
Opinion | Includes “Letter to the Editor” and “Advice” |
Review | Art, Literature, Film, and other reviews of existing media artifacts |
Event Announcement | Obituaries, Weddings, Performances, Scholarship Winners, etc |
Social Media | Includes Twitter, Reddit, and other forum/public posting sites |
Rhetorical/Structural Codes: | |
“Practical” vs. HLA (majors) | Any situation in which “practical” fields of study (STEM, Medicine, Business, Vocational certification, etc) is explicitly compared to/pitted against the humanities, or vice versa. The two fields often seek to define one another. These articles often have to do with student choice of major and enrollment, but are sometimes about the overarching ideology/methods/popularity of the fields as a whole—hence the parentheses around “(majors)”. Often occurs in abstract situations in which people debate/extol/decry the “versatility” of certain majors/skills vs. the specificity/narrowness of others |
Quantitative Data | Any invocation of statistics, large data sets, or other “numbers based” arguments or facts, with regard to money, people, or any other large data set |
Philosophy of Education | Debates and declarations about the purpose and form the humanities/the university ought to take. Within the discipline, this often takes to form of “old” vs. “new” methods. Otherwise, it often either takes the form of “HLA advocacy” (see About codes), or invokes a hard-nosed “run it like a business” structure that focuses on college as an investment. In the context of higher ed, it often manifests itself in terms of the liberal (arts) education model in traditional university vs. highly specialized programs of study, including private universities, community colleges, and certificates |
News | Events, occurences, programs, scandals, or other “fact-based” reporting such as that which constitutes a more traditional news article |
Narrative Framework | A first-person story or experience–something being “shared” as a narrative without necessarily arguing or advocating |
Argument/Debate | Any article whose primary purpose is making a case for/against a postion from the author’s POV, (rather than “reporting” on a particular event or policy). This Rhetorical code is particularly common in the “Social Media” genre |
“About” Codes: | |
Employability and Income | Employable skills, post-graduation jobs and income, and a “return on investment”. Desirability of income is included in this category |
HLA (Humanities and Liberal Arts) Advocacy | Articles that defend the humanities, liberal arts, or both; they argue for the continued relevance and necessity of a humanistic education |
PPR (Public Perception Research) | Broadly speaking, what “everyone” says, both with regard to large polls/numbers and to personal opinion/preference |
Policy and Politics | Government and university policy, as well as discussing/using education as a policy platform. Nearly always overlaps with but subtly different from “Funding” |
Extraeconomic Benefits | Sometimes overlaps with “CTCW”, but more often tends to be a bit more idealist: emphasizing empathy, a sense of different cultures, and the social responsibility of being active, global citizens. Benefits for the individual within society |
Funding | Dealing with public and private sources of funding, crises in funding, and public policy on funding, esp. with regard to higher education |
CTCW (Critical Thinking, Communication, and Writing) | A discussion of the importance of attention, synthesis, analysis, and rhetoric both within and outside the academy. Sometimes (but not always!) overlaps with “extraeconomic benefits” |
Higher Education | Articles dealing with college or university education practices, policy, or issues. In this category, I am including any post-secondary certification programs, as well as discussions of pedagogy/programming |
Student Life | In the context of education, this is a more social/personal take on the issues of access, study, extracurriculars, family support/conflict, and other subjective experiences that are separate from the more institutional/administrative concerns of “Higher Education” or “K-12” |
Arts Enacted | Similar to “Student Life”, but in an public rather than university context. Includes performances, gallery shows, protest art, concerts, etc. Political activism art–including “hacktivism” and creative political demonstrations–also apply |
Pro-Traditionalism | A relatively small category, but distinct enough to merit its own code. Almost always overlaps with “HLA advocacy” and “CTCW”, but often aligns itself against “Interdisciplinarity/New Methods”. A call for the importance of the liberal education model, the lecture format, library stacks, and/or in-depth attention and close-reading. Often, a rejection of scientific “encroachment”, technophilia, and “populist” learning models |
Interdisciplinary/New Methods | Articles detailing new, interdisciplinary, or innovative ways of talking about or practicing humanist research and discourse. Digital humanities, discourses bridging the sciences and the humanities, and new ways of thinking about old problems are all relevant to this category |
Crisis/Changing World | Emphases on how the world/academy/job market is changing drastically–as opposed to simply “fluctuating” with the times. This sometimes takes the form of “crisis” language, but also sometimes embraces the change. Essentially, this is any article that indicates a perceived ideological paradigm shift, rather than a single instance of something |
Engaged Citizenry | Similar to “Extraeconomic Benefits”, but more specifically focused on the civic duties/democratic awareness/social responsibility of large groups of people rather than a focus on the individual |
Social Justice | Questions of institutionalized in/accessibility, discussions of prejudice and intolerance against social minorities of all stripes (racism/sexism/classism/religion etc), knowledge (or lack thereof) about historical atrocities/broad societal patterns of prejudice |
K-12 | Articles dealing with K-12 lesson planning, policy, or other educational practices. This includes informal (i.e. “at home”) educational interaction with children, as well as children’s programming, museums, and events |
Conclusion
Although ultimately subjective in nature (Constructivist Grounded Theory was developed in the context of Sociology, and takes as a foundational assumption that the representation of data is inevitably situational, mutually constructed through iterative interaction between researcher and data–see “Constructivist Grounded Theory” for more details), a robust codebook and a small corpus of hand-coded articles helps a human peruser identify, at a glance, non-obvious rhetorical and content patterns in qualitative data sets. These patterns stem from substantive engagement with the raw data itself, but avoid getting bogged down with individual details. Essentially, it is a method for finding and analyzing widespread discourse patterns, building theory inductively rather than deductively (that is, avoiding the precritical application of a preexisting theory or framework). In other words, grounded theory methodology attempts to walk the line between claims of neutrality and confirmation bias–it is neither quantitative “big data” nor a verification method for established ideas. By using grounded theory to analyze discourse patterns within our 1BC before having a specific research question in mind, we hope to have provided the WE1S project with a starting point from which to tackle humanities research questions at big data scales.
Sources:
Charmaz, Kathy. Constructing Grounded Theory, 2nd ed. SAGE Publications, 2014 (original publication 2006).